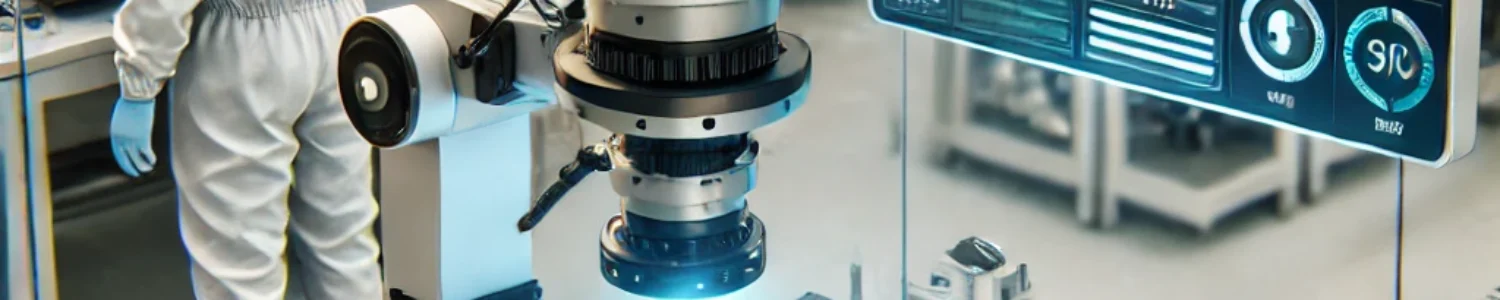
How AI and Machine Learning Are Revolutionizing Semiconductor Wafer Inspection
The semiconductor industry is at the heart of modern technology, powering everything from smartphones to artificial intelligence systems. However, as the demand for faster, smaller, and more efficient chips grows, so does the challenge of maintaining high yields in semiconductor manufacturing. One of the most critical steps in this process is wafer inspection, where defects must be detected and classified with extreme precision.
At Nogs CS, we specialize in developing AI-driven software solutions that enhance the defect detection process in semiconductor wafer inspection. By integrating machine learning, data analytics, and automation, we help manufacturers improve quality control, increase yield, and streamline operations.
The Growing Need for Smarter Wafer Inspection
As semiconductor technology advances, wafers are becoming more complex, with finer feature sizes and more intricate circuit designs. Traditional wafer inspection systems rely on optical and electron microscopy to detect defects, but these methods are increasingly insufficient due to:
- High False Positive Rates – Manual review of defects flagged by traditional systems slows down production.
- Inconsistent Defect Classification – Human judgment can lead to variability in identifying defects.
- Increasing Data Complexity – Modern semiconductor fabs generate vast amounts of inspection data that require real-time analysis.
This is where machine learning in defect detection plays a game-changing role.
How Machine Learning Improves Defect Detection
Machine learning algorithms can analyze wafer inspection images with greater speed and accuracy than traditional methods. Our AI-powered solutions at Nogs CS offer several advantages:
1. Automated Defect Recognition and Classification
Instead of relying on predefined rules, our deep learning models learn from thousands of past inspections, enabling them to:
✔ Identify defects with near-human accuracy.
✔ Reduce false positives by distinguishing real defects from harmless variations.
✔ Improve over time as they are exposed to more data.
2. Real-Time Analysis for Immediate Corrections
Time is critical in semiconductor manufacturing. Our AI solutions process wafer inspection data in real time, allowing manufacturers to:
✔ Detect issues before they impact production.
✔ Adjust process parameters on the fly to minimize waste.
✔ Speed up decision-making for engineers and technicians.
3. Integration with Existing Wafer Inspection Systems
One major advantage of AI-driven solutions is their ability to integrate seamlessly with existing wafer inspection equipment. At Nogs CS, we develop modular software that works with:
✔ Optical and electron microscopy-based inspection tools.
✔ Automated defect review (ADR) and automated defect classification (ADC) systems.
✔ Manufacturing execution systems (MES) for streamlined production workflows.
Beyond Inspection: How AI Helps Improve Semiconductor Yield
Defect detection is just one part of the equation. AI and predictive analytics also help manufacturers improve overall semiconductor yield by identifying defect trends and optimizing production parameters.
✔ Early Fault Detection – AI models can predict which wafers are likely to develop defects, allowing preventive action.
✔ Process Optimization – By analyzing defect distribution, manufacturers can adjust lithography, etching, and deposition processes for better results.
✔ Defect Root Cause Analysis – AI helps pinpoint the exact sources of defects, from contamination issues to lithography misalignment.
By implementing these AI-powered techniques, semiconductor fabs can reduce production costs and increase chip output, ensuring they stay competitive in an industry where efficiency is everything.
The Role of Cleanroom Technologies in Wafer Inspection
Wafer inspection doesn’t happen in isolation—it occurs in carefully controlled cleanroom environments, where even a single dust particle can ruin a chip. While cleanroom technologies have traditionally focused on environmental control (such as air filtration and temperature regulation), AI-driven software is now playing a role in:
✔ Contamination Detection – AI-powered image analysis can detect tiny contaminants that may lead to defects.
✔ Environmental Monitoring – Sensors collect real-time data on cleanroom conditions, which AI uses to predict contamination risks.
✔ Robotic Handling Optimization – Automated wafer-handling systems use AI to reduce human-induced contamination during inspections.
At Nogs CS, we work with semiconductor manufacturers to develop cleanroom-compatible AI solutions that integrate defect detection, environmental monitoring, and process control into a unified system.